Fleet Electrification Knowledge Center
The electric fleet journey, guided by a global leader in connected vehicles
Get insights to goGoing Electric
Fleet electrification is a gradual process. Learn how EVs can help you stay compliant, reduce costs and meet sustainability targets. Start planning your own EV fleet adoption strategy today.
Operating Electric
Adding EVs to your fleet is only the first step. Learn how to optimize your operations to get the most out of your investment.
The complete fleet electrification roadmap
Your new step-by-step guide across the full fleet electrification journey, including:
• Electric vehicle benefits for fleets
• EV deployment for different fleets
• Choosing the right EVs and charging stations
• How to optimize EV fleet operations
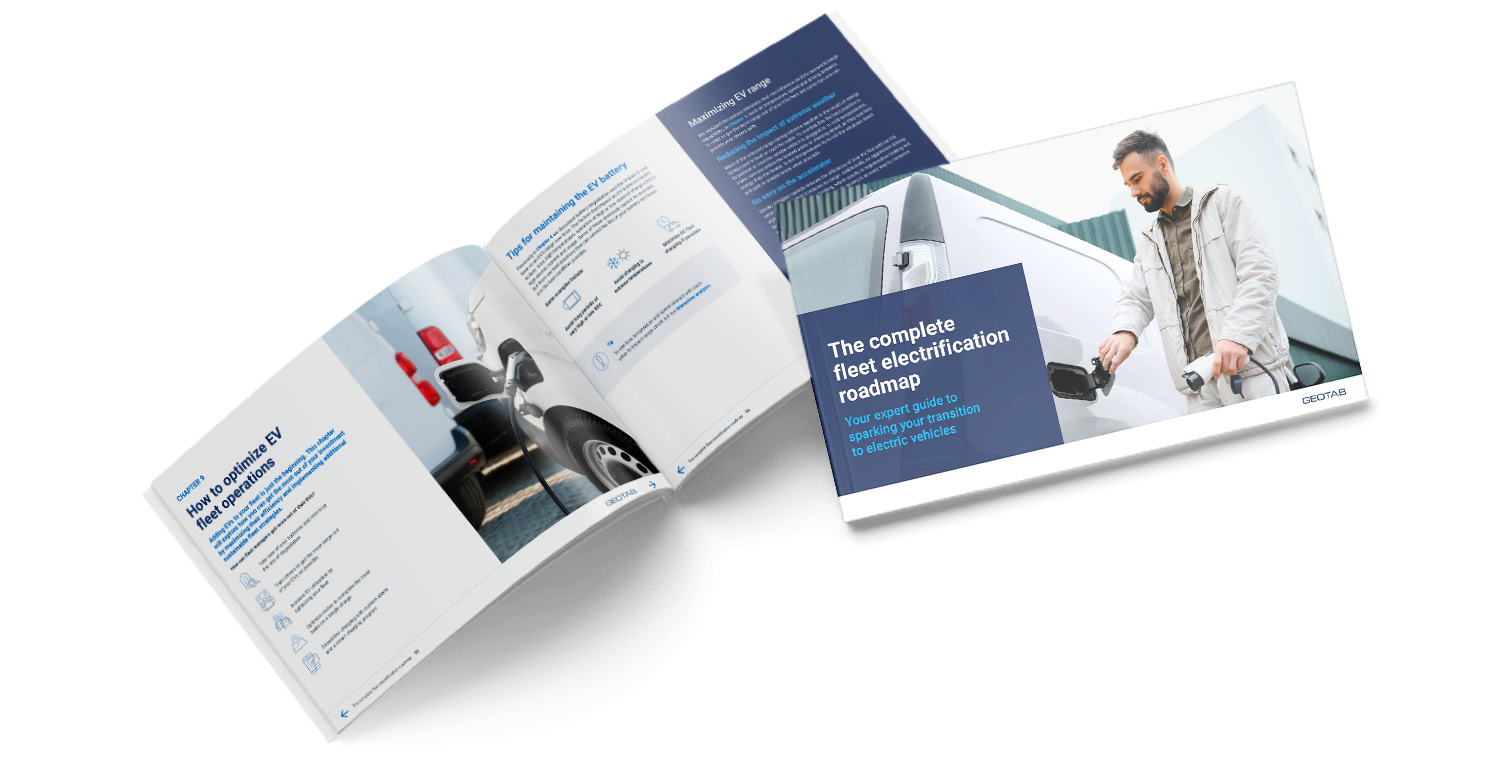
Spark your research with our free ebook today
Try our interactive EV Analyses
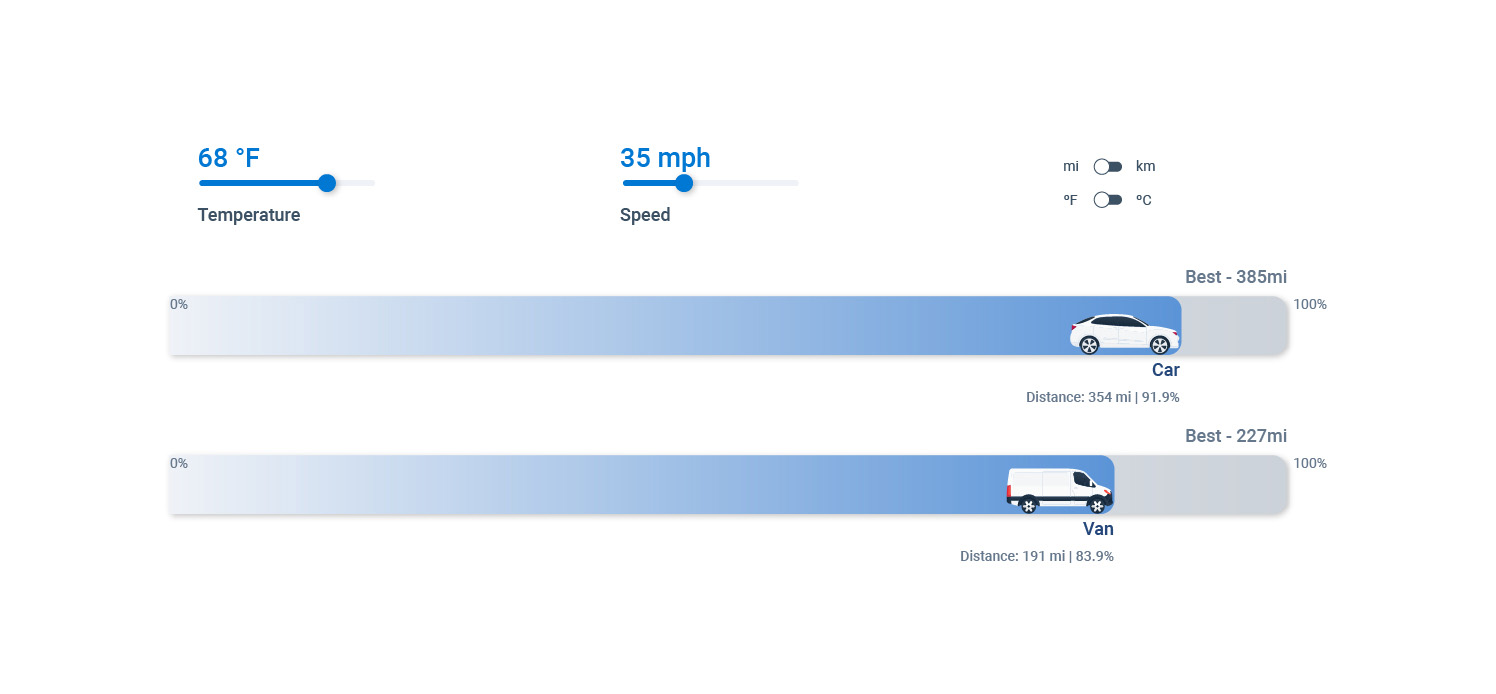
Analysis
Understand real-world EV range impacts
Can EVs meet range requirements? This analysis is based on real-world data from over 3 million EV trips and shows you how temperature and speed can affect how far an EV can drive on a full charge.
Learn more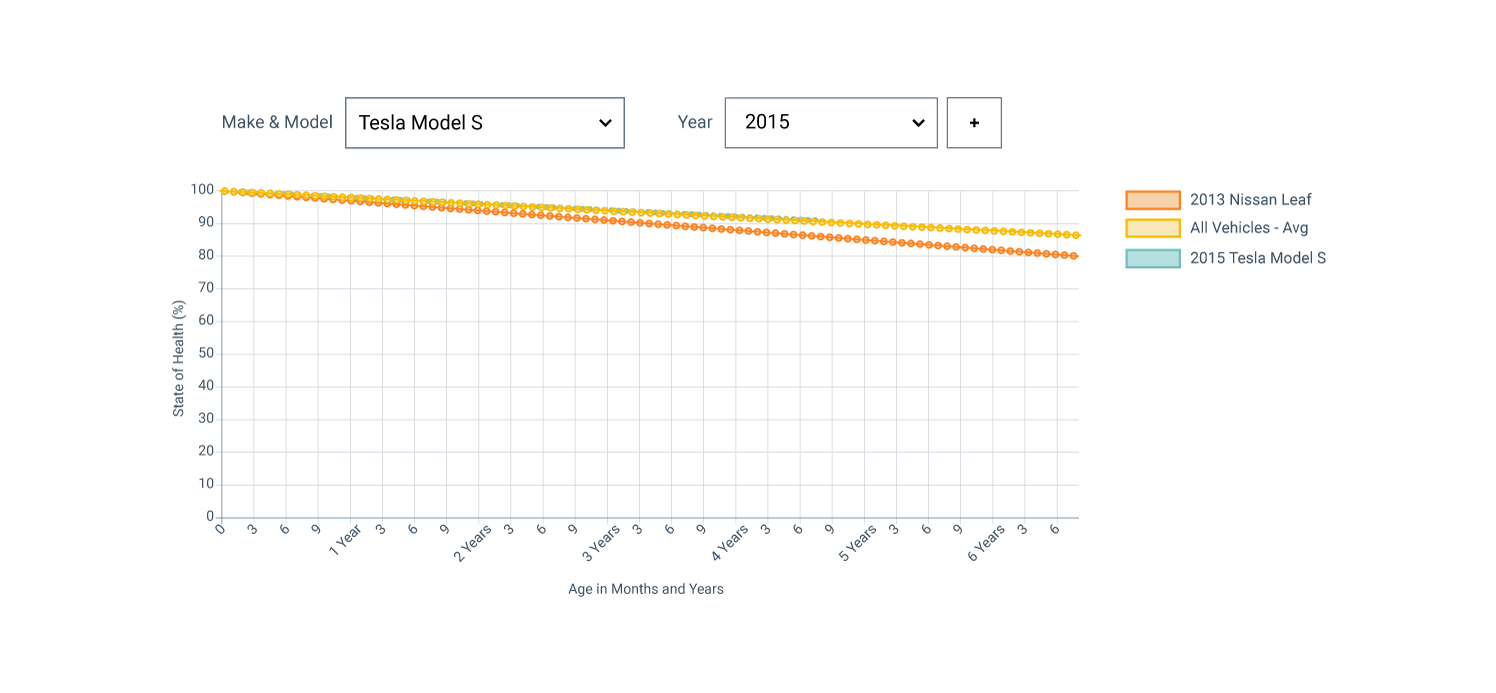
Analysis
Determine an EV battery's lifespan
Understand the rate at which EV batteries degrade and gain confidence in your decision to go electric. This analysis lets you compare battery degradation over time for different vehicle makes and models.
Learn moreTry our EV Suitability Assessment tool
Create an electrification blueprint with projected environmental and cost savings