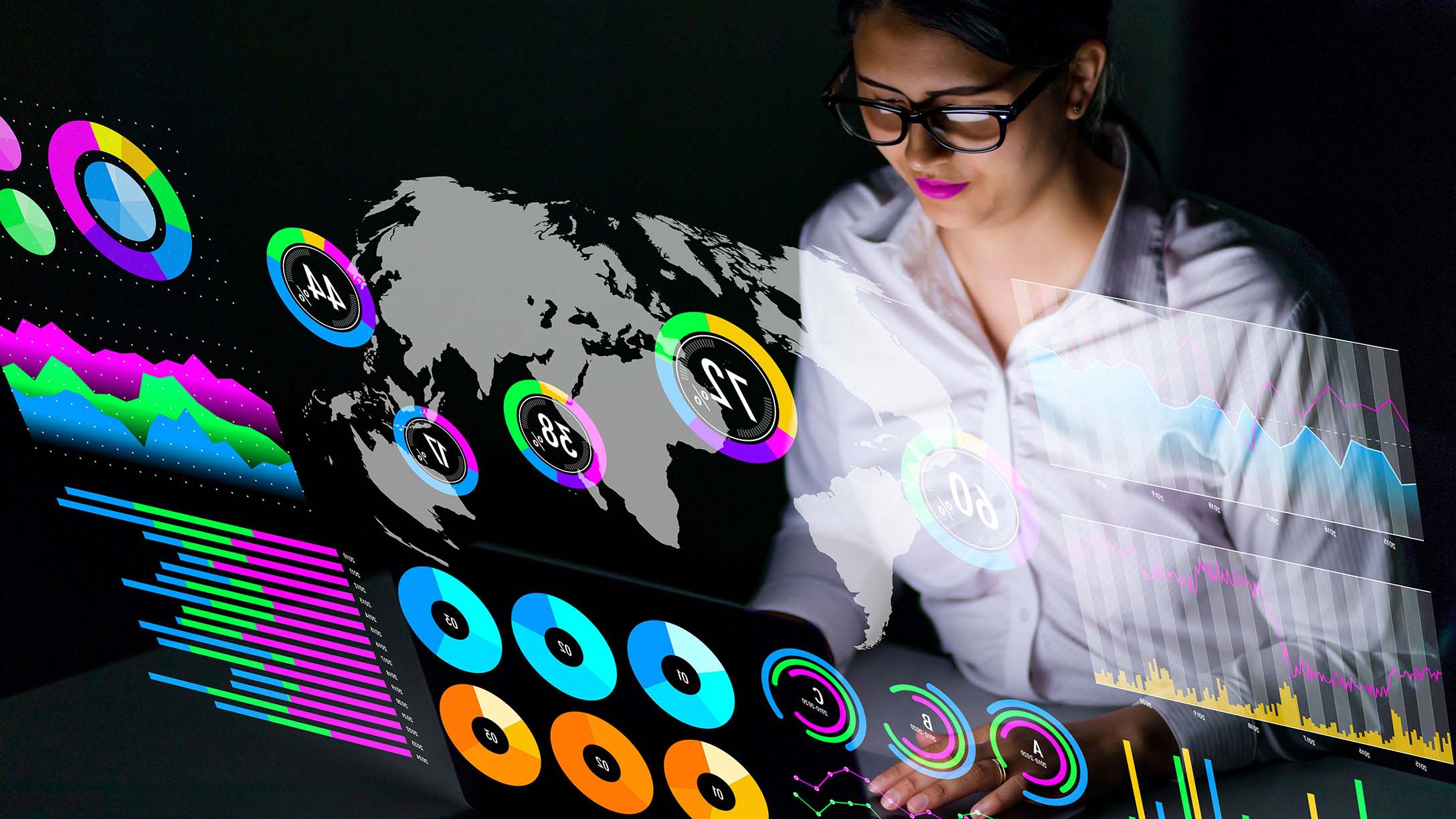
Don’t make these big data mistakes
Last updated on April 24, 2023 in Smart City by Paul Fabbroni | 4 minute read
Table of contents
Advice from a Geotab software developer.
The key to big data isn’t the amount of data that you have, but what you do with it. If you have joined the big data revolution, make sure you avoid these top big data mistakes.
What is big data?
What do we mean by “big data?” Big data refers to the large volume of data that businesses receive on a daily basis. This data can come from sources such as transactions, marketing campaigns, social media, streaming devices, scanners and others. Big data can also come in various forms such as structured numerical data, unstructured text, video, audio and so on.
It can be overwhelming to make sense of data and turn it into something useful. This is why companies may end up with graveyards of unused data. But harnessed correctly, big data can be an extremely powerful tool that helps companies reduce costs, increase productivity, improve decision making, and most importantly — increase revenue.
See also: Big data glossary
Big mistakes with big data
It’s clear that leveraging big data can be greatly beneficial to organizations, but many aren’t sure where to start. Here are 10 mistakes to avoid for companies looking to start a big data initiative.
1. Don’t work in isolation.
Different teams bring their own insights, so involving all the business units in a big data project can prove to be valuable. The data and analytics team can extract the data while IT sets up the infrastructure to store and maintain the information. The sales team might have the key to explaining an anomaly, and the marketing team can help promote your business and drive sales. Working with different teams will help you leverage your data to the fullest.
2. Don’t start big.
Do not apply the phrase, “go big or go home” right away. Before jumping on board with big data and trying to use it on your exciting new venture, try using it to prove something you’ve already learned from one of your smaller projects. Then, apply what you’ve learned about big data to a large-scale project. The experience you gain from practicing with activities you are familiar with will allow you to optimize your predictive models for use with bigger projects.
3. Don’t forget about data security.
When data is aggregated, it is grouped by similar attributes and no individual data is shown. However, once you navigate through the data, you need to ensure that it is private and secure in order to protect your company from data privacy violations. In addition, if you are receiving third-party data, you need to verify that you are allowed to use this data as a part of your analysis before beginning any projects.
4. Don’t assume anything.
There are various software packages available for data analytics, so it is important to take the time to investigate which one is right for what you are trying to accomplish. Keep in mind that data analytics may be just one part of your analysis. Several other types of analytics may be useful to you as well, such as text, predictive and spatial data. It is unlikely that one package will cover all your needs.
5. Don’t disregard small data.
Once you have successfully implemented big data, do not think you no longer need the “small data.” Your existing data is customized to your business’ needs and contains benchmarks and business rules that you can use to compare against your big data.
Quick Tip: Use your data warehouse in conjunction with big data to help fill in the gaps and provide a complete story of what the data is telling you.
6. Don’t forget to confirm consistency.
Metadata is used to describe the content and characteristics of other data. Once you have analyzed your existing data and come up with a set of metadata for it, you need to ensure that the new incoming data is cleansed and adheres before you can trust it. Incoming data will have various formats and structure from one source to the next, so you may need to check its consistency and confirm the validity. How do you do this? Repetitive observation and analysis is required before you can use the data.
7. Don’t overlook the importance of a cloud storage provider.
Big data deals with petabytes of data (one petabyte is equal to one million gigabytes) which is an enormous amount of data. Deciding on how and where to store this data is something to consider when starting a big data initiative. Depending on how often you access the data, the size of the data set returned, hardware costs and memory, the costs can vary greatly.
8. Don’t overlook execution.
While big data can be used to create visually appealing and easy to understand charts and graphs, the computation of data to produce these representations can take a long time. Take your time to come up with a strategy for managing your data’s performance. This is key to the success of your project as even the most useful graphs can render an application useless if it takes five minutes to load.
Depending on your application, it may be possible to use snapshots of your data that are pre-generated at frequent intervals rather than real time. You should also come up with a set of standards and naming conventions to make sure your data structure is consistent and optimized.
9. Don’t focus on one blanket solution.
Similar to selecting a software package, don’t assume you can apply the same method of predictive analysis to all parts of the business. Marketing, sales, HR, finance and IT can all have very different goals or problems you are trying to solve and may require human interaction in order to fully understand and make use of the data.
Try to focus on improving and automating one routine decision process at a time for each department rather than trying to apply a blanket analysis for everything.
10. Don’t focus on data collection.
Instead of focusing on collecting as much data as possible, focus on collecting useful data and figuring out how to apply it toward the business processes you are trying to fix.
According to the Digital Universe study, only three percent of the 2.8 zettabytes (one zettabyte is one trillion gigabytes) of data available was ready for manipulation, and only 0.5 percent was used for analytics. Predictive analytics can help with knowing what data to collect and how to apply it to the current business processes.
Conclusion
In 2013, Viktor Mayer-Schonberger and Kenneth Cukier wrote a book entitled “Big Data – A revolution that will transform how we live, work, and think”, and we can see today that this is already becoming true. Like Facebook, Google, and Instagram, most major websites and apps are constantly collecting data based on user interactions and using it to customize the ads and preferences. Big data is also being used by companies like Geotab to help make cities safer by identifying hazardous driving areas, potholes and areas that have a high probability of accidents.
The applications of big data are endless, figuring out how it can help and how to apply it to your organization is up to you, but avoid these common mistakes!
Subscribe to the blog for more stories like this.
More stories from the Data and Analytics team:
New Study: Be Alert to Moose Car Crash Risk
Google Cloud Next: Geotab at the Forefront of AI for Smart Cities
If you liked this post, let us know!
Disclaimer
Geotab's blog posts are intended to provide information and encourage discussion on topics of interest to the telematics community at large. Geotab is not providing technical, professional or legal advice through these blog posts. While every effort has been made to ensure the information in this blog post is timely and accurate, errors and omissions may occur, and the information presented here may become out-of-date with the passage of time.
Get industry tips and insights
Sign up for monthly news and tips from our award-winning fleet management blog. You can unsubscribe at any time.
Republish this article for free
Other posts you might like
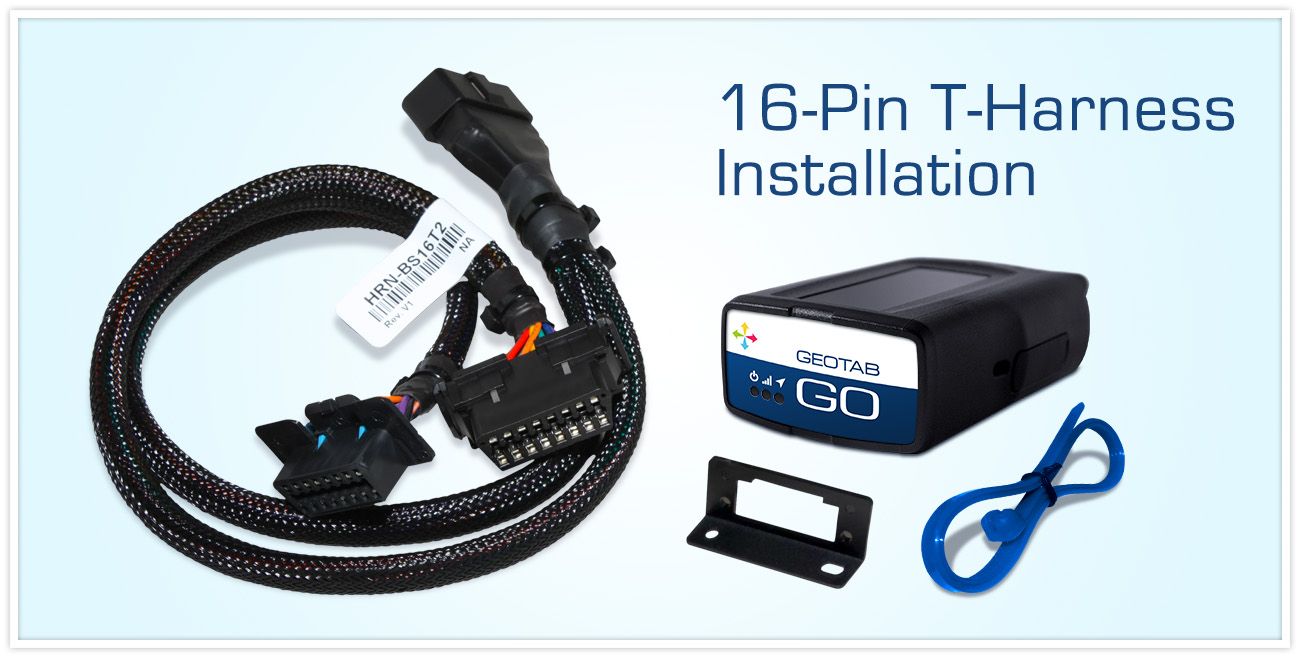
Geotab’s 16-pin t-harness fleet management device
November 30, 2023
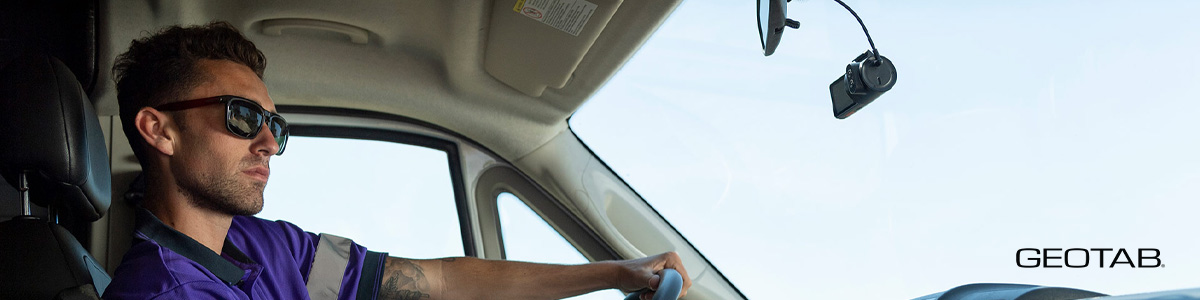
Video telematics: Safeguarding the future of fleets in Mexico |Geotab
October 25, 2023
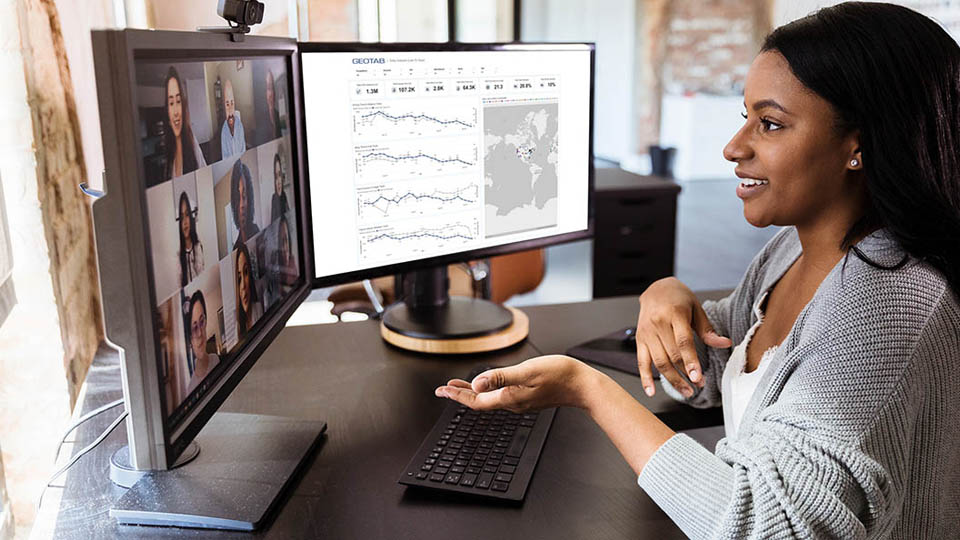
Unlock the power of your data with Geotab Data Connector
March 1, 2023
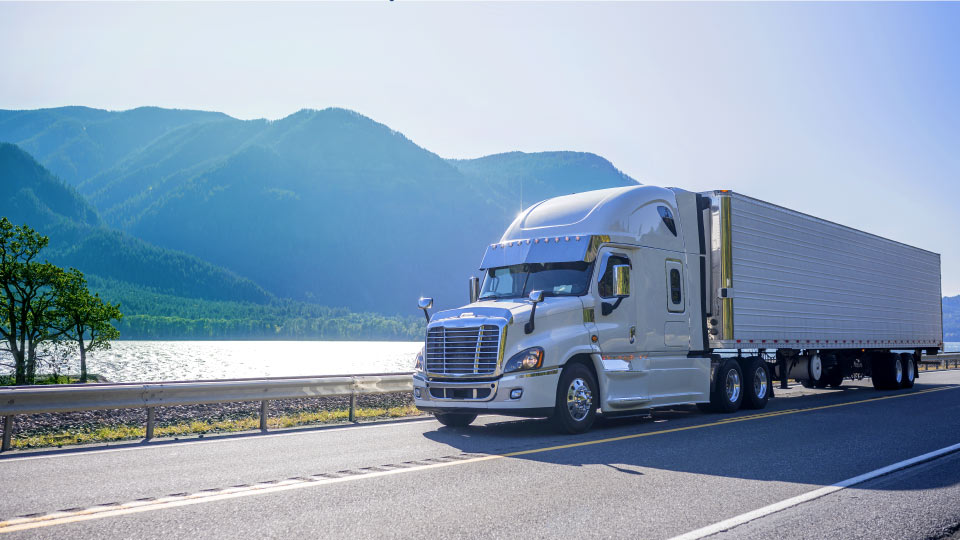
The top 5 ways fleets use data and telematics to succeed
February 17, 2023