The impact of artificial intelligence on the mobility industry
What will be the impact of artificial intelligence (AI) on jobs and the mobility industry? Read why AI is relevant to your future.
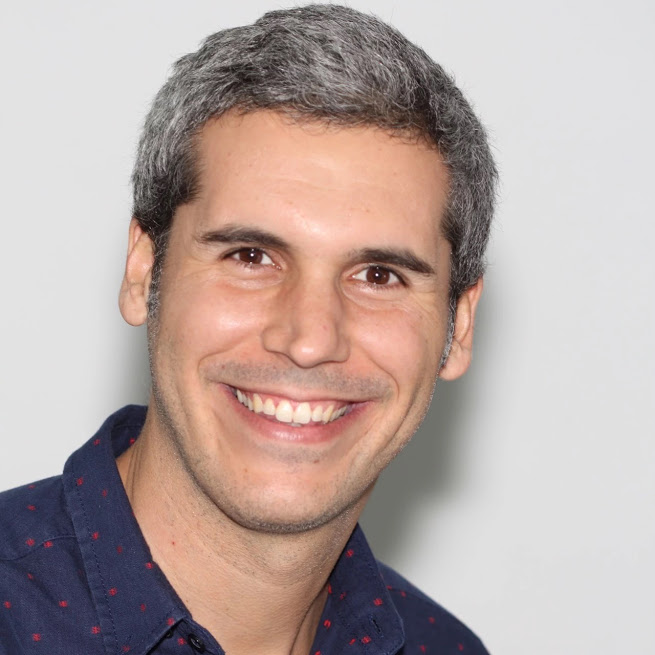
By Ivan Lequerica
Vice President, South Western Europe at Geotab
Dec 29, 2017
Updated: Apr 19, 2023
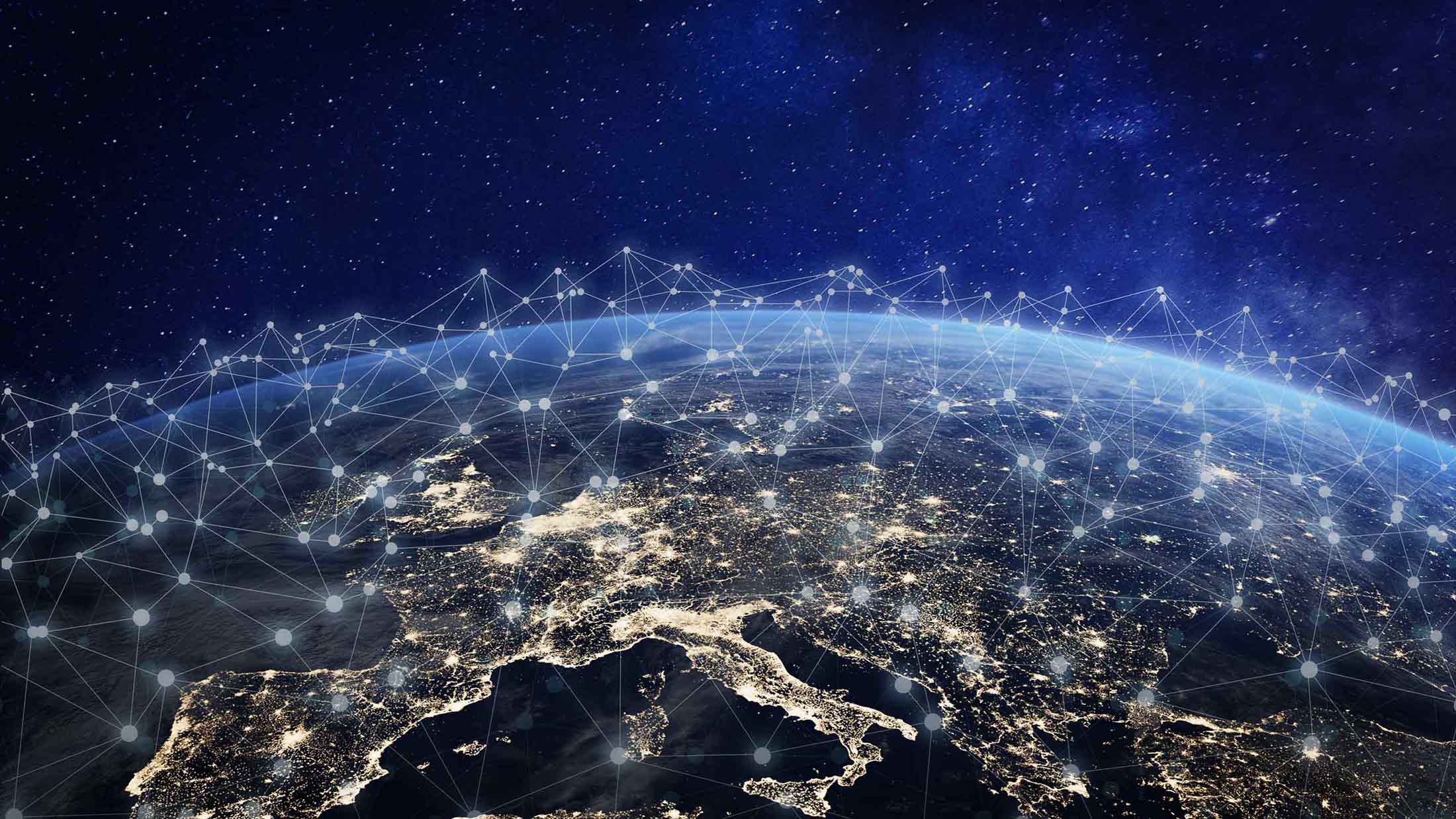
It is clear and well accepted that artificial intelligence (AI) will change our lives, but uncertain about when this will happen. Leveraging these technologies will allow companies as individuals to do more tasks with less efforts, automating complex processes. This article explores the impact of artificial intelligence on the mobility industry.
See also: Boost day-to-day fleet reliability with AI
What is artificial intelligence and why is it relevant?
Defined in 1956 by Professor John McCarthy, artificial intelligence is “the science and engineering of making intelligent machines, especially intelligent computer programs.”1 A subset of AI is machine learning — described as the field of study that gives computers the ability to learn without being explicitly programmed.
According to McKinsey, the application of AI will enable productivity growth and other benefits not just for businesses, but also for entire economies. Estimations from a macroeconomic point of view indicate that automation alone could increase productivity growth on a global basis from 0.8% to 1.4% every year.
AI will have a similar transformative impact as electricity had 100 years ago. It will change every single industry from healthcare to transportation, communications and manufacturing.
Although the idea of AI is not new, the pace of recent breakthroughs definitely is. There are three main factors fostering this acceleration:
- Computing Capacity — Advances are emerging beyond the current generation of central processing unit (CPUs) and Graphics processing units (GPUs). This capacity has been aggregated in high scalable data centers and is accessible through the cloud.
- Big Data — Huge amounts of information (images, voice, video, location, sensor information…) are collected through IoT processes and can be used to train AI models.
- Machine Learning (ML) algorithms have advanced significantly through the development of deep learning based on neural networks. Also the accessibility of these algorithms via toolkits like scikit-learn and Tensorflow is helping developers and data scientists.
Related: What Is Machine Learning?
AI and technology adoption
Companies will need to keep in mind a number of relevant considerations before getting started with AI. The technology is complex and its success depends on the environment (i.e. data quality, company culture or customer appetite). Typically, AI has some impact on the transparency of the decisions and the General Data Protection Regulation (GDPR), which requires companies to clearly state what they do with the data, may add extra complexity.
There are also significant concerns on security, ensuring that an AI is not either hacked or programmed maliciously must be taken into account in early phases. While speaking in Toronto, an emerging global hub for AI, Canada’s Prime minister Justin Trudeau reinforced the importance of ethics applied to AI and suggested a task force in Canada where there is a strong moral and ethical code that welcomes diversity.
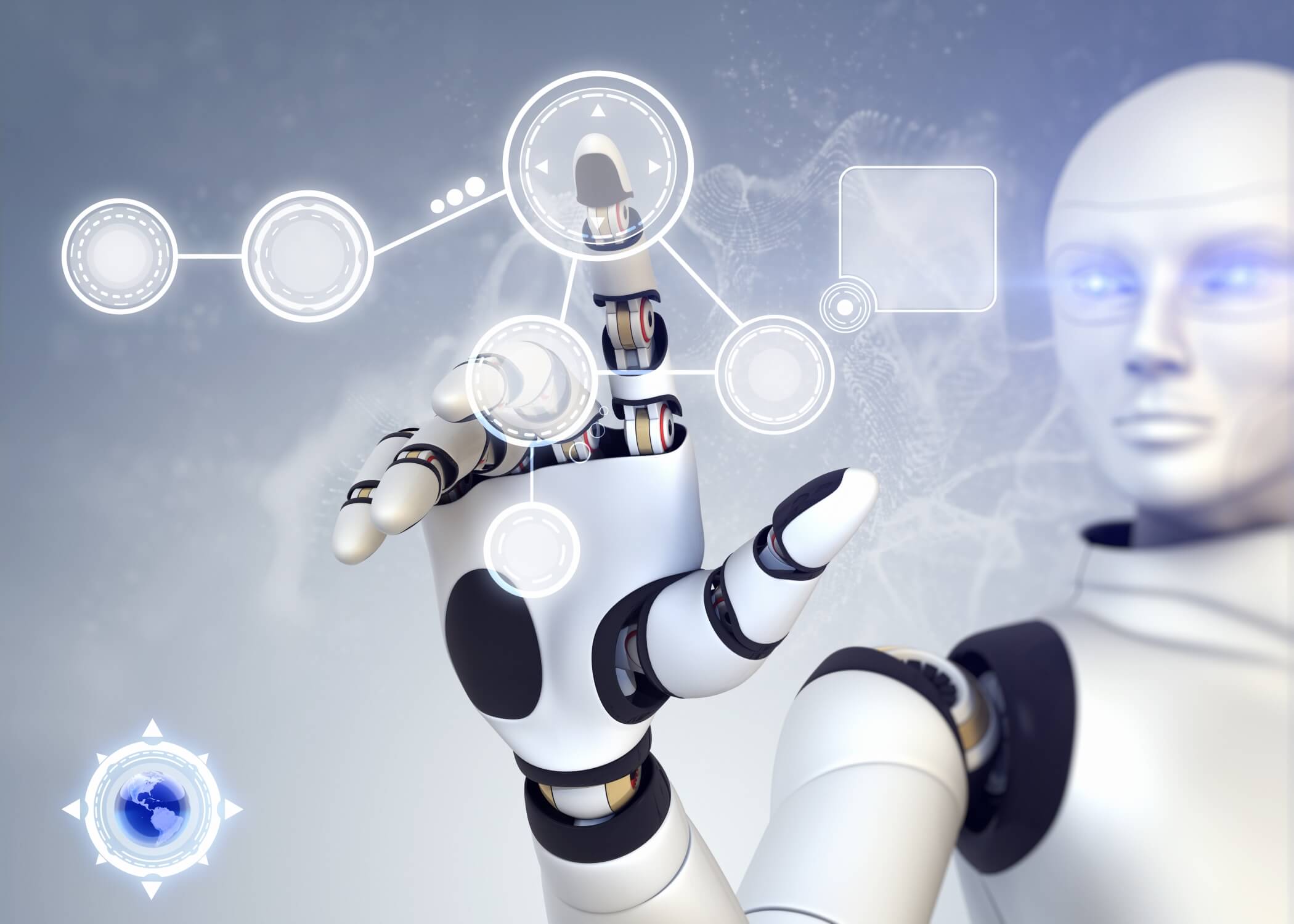
What is the impact of artificial intelligence on jobs?
An interesting debate is centered around how human occupations will be affected. McKinsey reports that only a small proportion of all occupations (about 5%) are fully automatable using current AI technologies, while 60% of occupations have at least 30% of the activities that can be automatized. This applies also to CEOs, since they spend about one quarter of their time on activities that machines could do, like analyzing reports and data to inform decisions.
Rather than replace jobs, AI will reduce some tasks in many professions and workers will have more time to focus on activities that adds more value. By removing less creative tasks from their work, AI can actually increase job satisfaction of employees.
AI applications in the mobility space
AI and machine learning technologies will have a significant impact on the automotive and mobility industries as they will bring new products and business models rather than only productivity improvements.
According to Microsoft, by the year 2025, 100% of new cars will be connected and by 2030, 15% of new cars will be autonomous — sending, receiving and analyzing “vast amounts of data.”
Cars are data centers on wheels. All that information can be used by many stakeholders (traffic authorities, leasing companies, municipalities, vehicle manufacturers, insurers, workshops, emergency services, etc.) in the mobility space to improve processes.
Some examples of AI in mobility:
- Accident reduction — ML techniques will learn what are the different causes of road accidents; distraction, weather conditions, fatigue, vehicle failure… Having IoT sensors on-board we’ll be able to understand in depth the reasons behind an accident and also to predict when a similar accident can occur again (with reasonable probability) and warn the driver and the company, improving fleet safety.
- On demand public transportation will consider both historical and real time data to plan routes according to the demand and capacity. Systems that all “talk” to each other — combined support improved routing and traffic patterns.
- Predictive maintenance algorithms will detect a potential failure based on statistical data from same models with similar driving conditions and schedule a workshop automatically attending the owner’s agenda.
- Intelligent logistics — Tracking operator patterns (order picking, inventory monitoring, and field force) combined with predictive orders in certain areas can minimize the delivery times and optimise stock levels at warehouses.
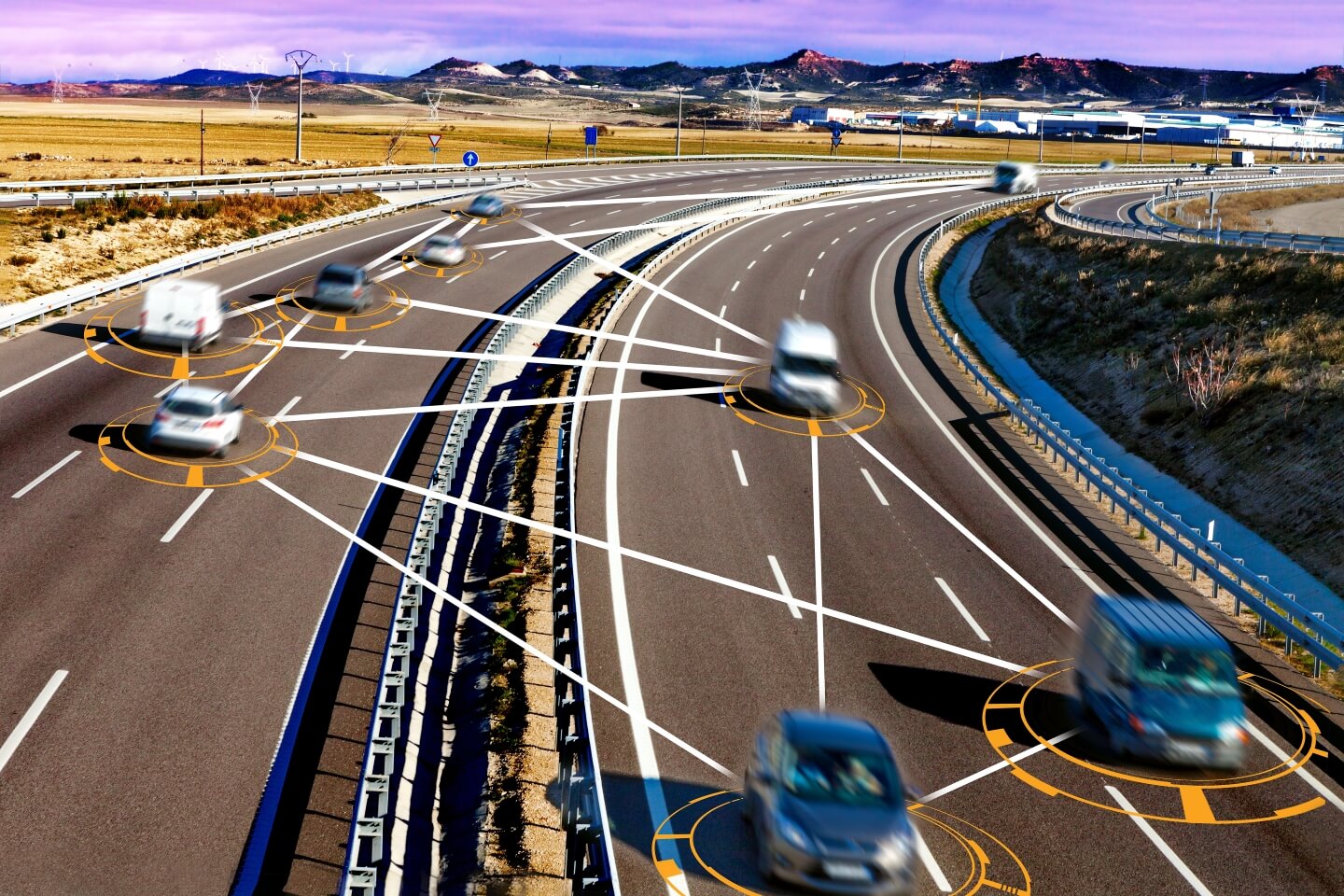
Geotab and AI: Rich data to build new tools
Geotab understands the potential of the AI applied to vast amount of data we collect daily (over 2 billion data points per day) and we are taking several steps forward to improve our processes and products using big data and ML techniques. Some concrete examples and initiatives are:
- Use data analytics to optimize operations in all our departments, forecast production, finance information, support calls, server logins, etc.
- Predict vehicle electrical problems. Considering battery voltage curves and real battery drain detections, we have developed a ML model able to predict when a battery is about to fail.
- Offer rich datasets. After aggregating and anonymizing datasets, we will be offering our refined data to third-parties for building valuable applications for the different industries. To mention some examples for smart cities:
Road impediments (potholes), detected with our device accelerometer help municipalities to locate where they need to repair the pavement.
Dangerous areas. Identifying areas in which accidents occur or where there are many harsh events help authorities to improve traffic signs.
Outside temperature information collected from vehicle computers to detect potential pollution areas and to predict more accurately weather conditions within a city.
Circling for parking. Identifying patterns of vehicles looking for parking slots will help infrastructure managers to plan public transportation and/or new parking areas.
Intersection traffic. Accurately measure traffic flows, including waiting times, identifying the type of vehicle at the same time (i.e. trucks vs passenger cars)
See what’s possible with telematics data: Read our smart cities white paper.
References:
- AISB. (n.d.). What is Artificial Intelligence. [Online]. Available: http://www.aisb.org.uk/public-engagement/what-is-ai
Subscribe to get industry tips and insights
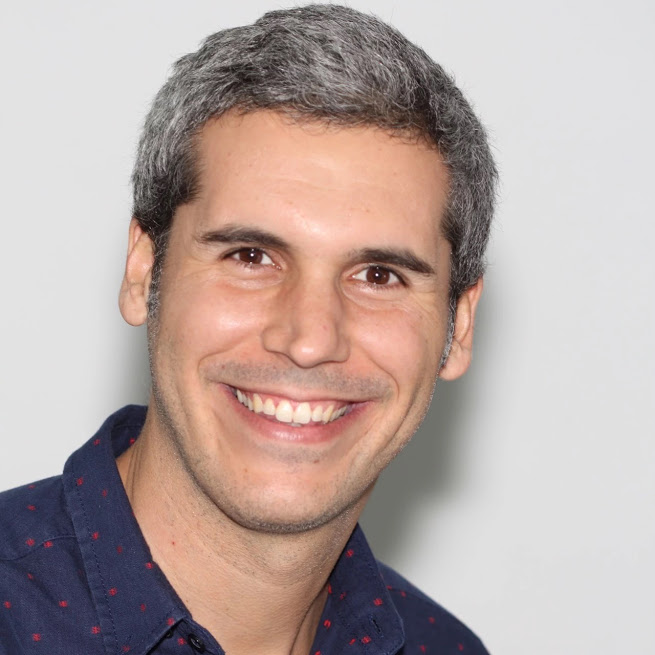
Ivan Lequerica
Vice President, South Western Europe at Geotab
Ivan Lequerica is a Physicist and Electronics Engineer PhD who has contributed to the development and implementation of Geotab’s European business and engineering strategies since he joined the company in early 2015.
Table of Contents
Subscribe to get industry tips and insights
Related posts
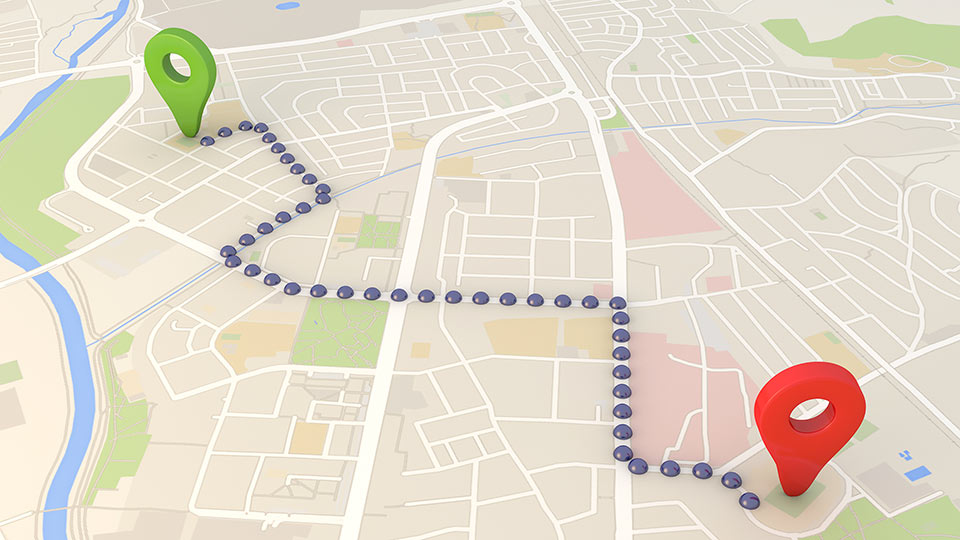
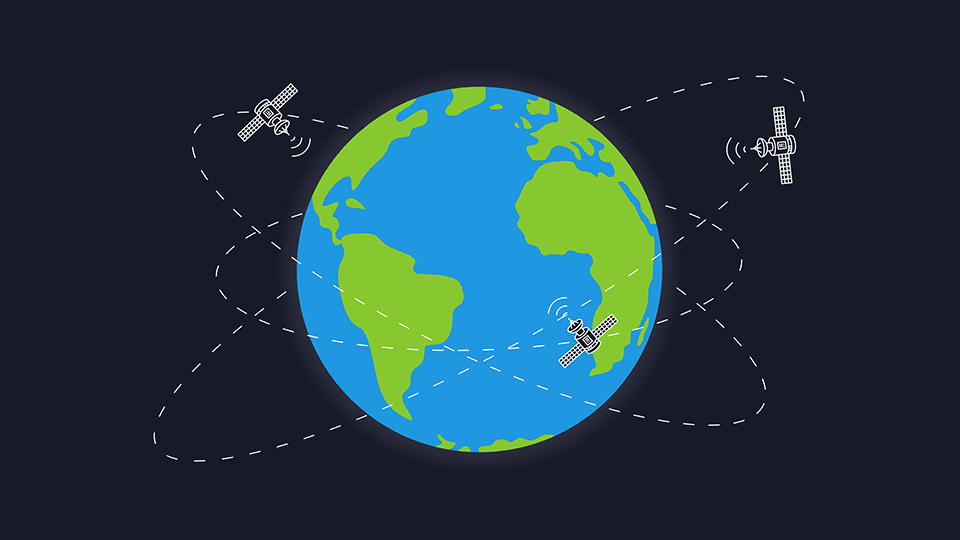
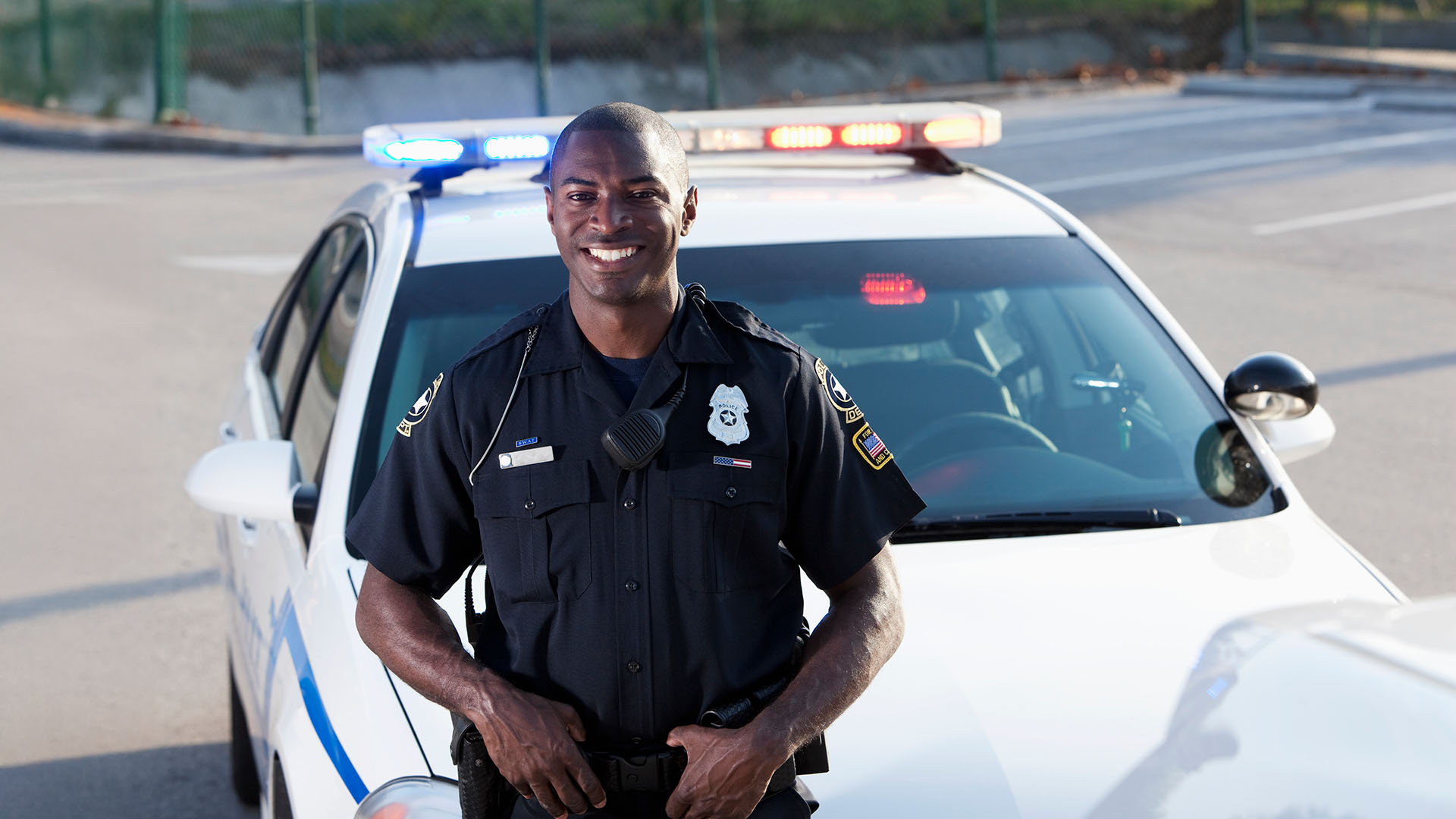
The impact of unproductive idling on police vehicle service life
June 10, 2025
3 minute read
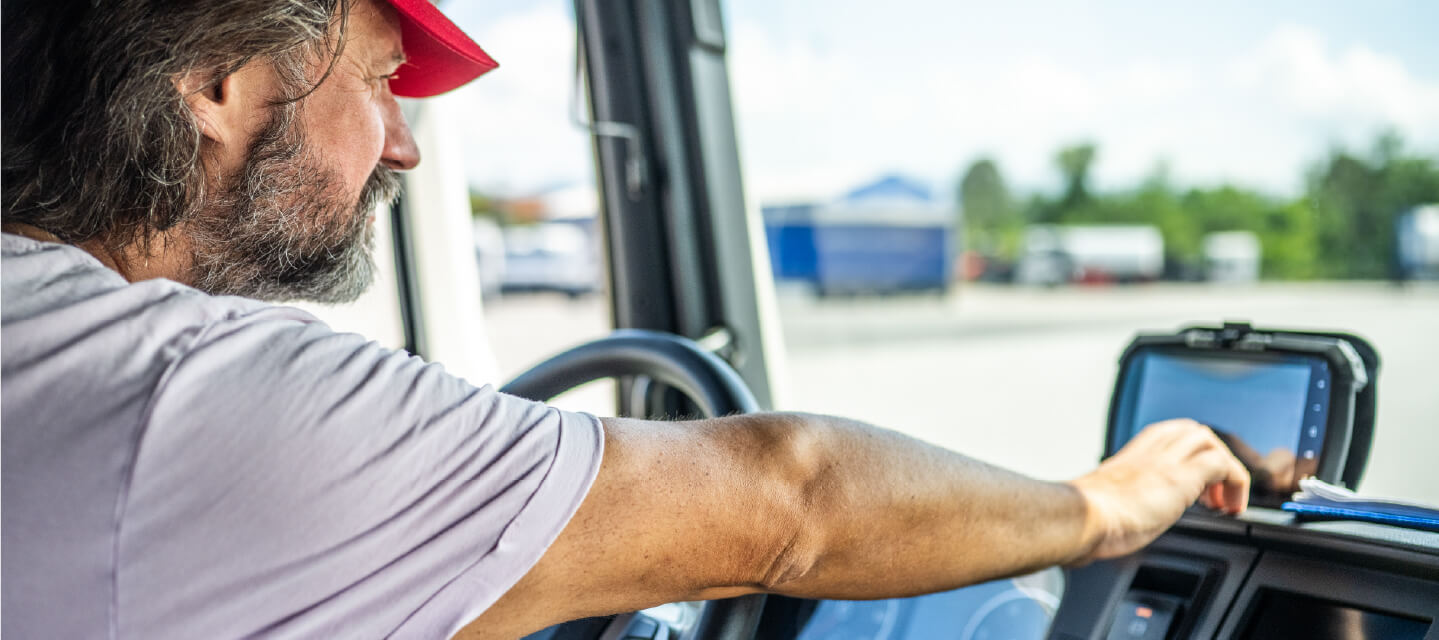
Multi-stop route planners: A fleet manager's guide + best tools in 2025
June 5, 2025
5 minute read
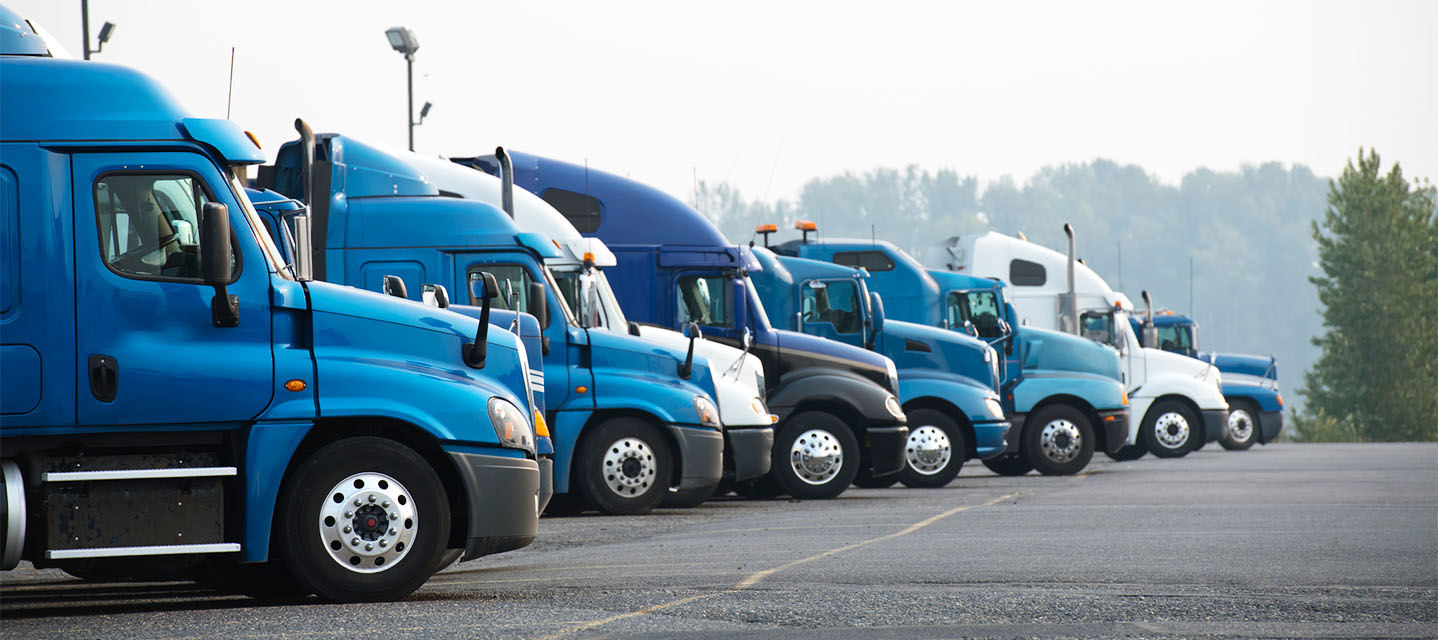
Commercial truck insurance cost: Rates by state + how to save
June 5, 2025
5 minute read